Finding Home: Social Matchmaking Through Machine Learning
- Team
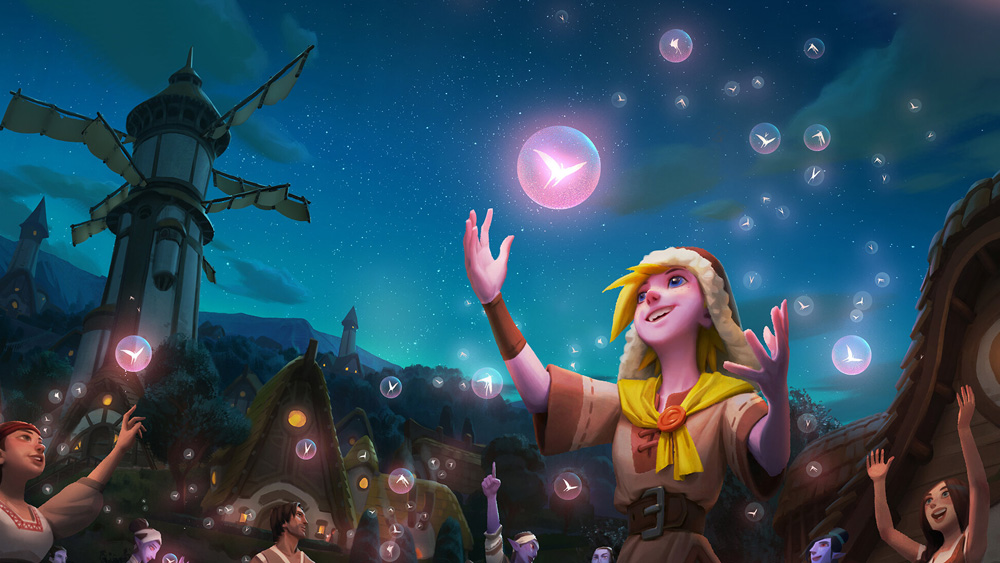
I grew up in a small town - so small that it technically qualifies as a village. To describe this to others, I quote my father’s favorite fact: “there are thirty times more cows here than there are people.” Depending on who you are, that may or may not sound appealing. Yet, another aspect of this community was more universally captivating - the feeling of being in a place where you truly belong.
As my education and career progressed, I made my way up the chain from cities to the dog-eat-dog world of metropolises. With each move, that strong sense of community support and inclusion in my hometown slowly faded away. It was easy to get lost in the millions in these larger places and feel like another number.
Online user bases, such as those present in video games, can quickly reach a population in the tens to hundreds of millions, surpassing the largest cities’ size. One of our goals at Singularity 6 is to create a world that brings us closer together, not further apart, which makes solving the large-scale problem of “community” vital to us.
"How do we design a social experience for millions of players while also connecting them in meaningful ways?"
In video games, the exploration of in-depth algorithmic solutions to optimize the social experience remains limited. However, the problem has not gone entirely untouched, as many social media platforms leverage services with machine learning components to solve similar problems. With a few modifications, these systems should work in the gaming space as well.
For example, link prediction, the problem of predicting a link between two entities in a network, is a standard tool for increasing connectedness in networks; if a system can accurately assist a user in finding relevant acquaintances, their likelihood of continuing to use the service increases. Unfortunately, the problem is somewhat different and more difficult in the gaming space; players are often anonymous and, if playing socially, generally choose to play with an explicit group of friends. In other words, the connectedness of the entire player graph is sparse compared to an average network.
In this case, the problem is partially about predicting those existing relationships and more about curating new ones. Can we learn the interactions that create a positive perception of other players? Can we incentivize our system to reinforce those positive interactions by increasing the chance that those players will meet again soon? With time, we can ensure that each player is automatically added to the best community for them when returning to the game.
"The problem is partially about predicting those existing relationships and more about curating new one."
While that task itself may sound daunting, there is good news on the business front. For some social apps (i.e., dating), a conflict arises with exactly how good a matchmaking service should be. If you build a well-optimized matchmaking service, it may hinder user retention, and if you throw together a scrappy solution, you may not get users in the first place. For social gaming, this trade-off between user experience and business performance is mostly absent. Without that tension, we can build a high-quality matchmaking service that will strengthen player bonds and impact the business in a meaningful way. Finding win-wins such as this is a motivating force for everyone here at Singularity 6.
For me, I hope to rekindle that sense of community I lost long ago and share that experience with others. I want to help build that safe, secure space that anyone can go to when they feel alone or when the world is a bit too chaotic. I invite you to follow Singularity 6 on this journey, the best one of all, the one of finding home.
-Riley Howsden, Machine Learning